Kozinsky and team among finalists for Gordon Bell Prize
Researchers recognized for equivariant neural network models that can quickly and accurately simulate millions of atoms
By Leah Burrows
October 5, 2023
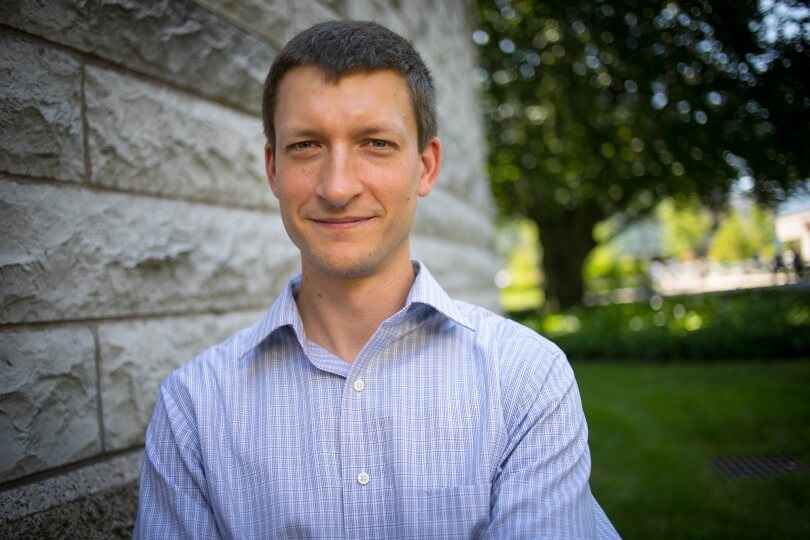
Large and atomistic are not words that typically go together but the systems that Harvard John A. Paulson School of Engineering and Applied Sciences (SEAS) Professor Boris Kozinsky and his team are most interested in are both— large and complex systems composed of millions or even billions of atoms.
Understanding the dynamics of these systems—which can include the building blocks of viruses or the surfaces of catalytic materials—can lead to breakthroughs in drug development, green energy and chemistry, organic electronics and more. But simulating large-scale structures on the level of atoms typically requires massive computational power and there has always been a tradeoff between speed and accuracy.
Kozinsky, the Thomas D. Cabot Associate Professor of Computational Materials Science at SEAS, and his team are breaking down that tradeoff, pushing the boundaries of what is possible in atomistic simulations.
The researchers developed a neural network architecture that can both quickly perform these so-called molecular dynamics simulations and do so at unprecedented levels of complexity and with quantum accuracy. Named Allegro for its speed and precision, this neural network was able to capture the motion of millions of atoms several orders of magnitude faster than previous methods.
For this work, Kozinsky and his team have been named among the six finalists for the Gordon Bell Prize, the most prestigious award in the field of supercomputing, given annually by the Association for Computing Machinery (ACM). The winner will be named this November at The International Conference for High Performance Computing, Networking, Storage, and Analysis in Denver, CO.
The prize recognizes the most valuable scientific computation demonstrated using state-of-the-art software and hardware technologies on world-leading supercomputers. Allegro's large-scale simulations were demonstrated on the Perlmutter supercomputer at the National Energy Research Scientific Computing Center of the United States Department of Energy.
The nominated team includes members of Kozinsky's lab, the Harvard Materials Intelligence Research group: Albert Musaelian, PhD '23, Anders Johansson and Simon Batzner, PhD '23.
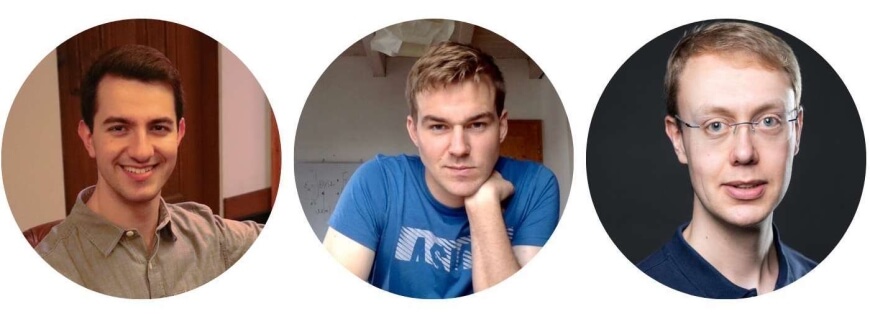
"Even the simplest life forms, like viruses, are incredibly complex, and contain many millions of atoms," said Kozinsky. "Being able to understand how these complex systems evolve over time and have faithful simulations of the kinetics of chemical and biological processes is important for applications in not only medicine but also chemistry, materials science and so much more."
Allegro addresses the tradeoff between speed and accuracy by combining the precision of so-called equivariant machine learning, which enables models to accurately learn the quantum interactions of large groups of atoms directly from their geometry, with a novel architectural design that enables massive parallelization and efficient GPU utilization.
The research team demonstrated Allegro's capabilities by performing extensive tests of computational scaling across different numbers of GPUs, running nanoseconds-long simulations of protein dynamics, and establishing the ability of the method to scale to a 44-million atom structure of a complete HIV capsid. Viral capsids, the protective shells that surround the virus's RNA, are a promising target for treatment and vaccination.
"These results show how Allegro can, at the same time, scale up equivariant methods in the amount of data used, the speed of simulations, and the size of systems. But maybe more importantly, they also show how it combines fundamental machine learning developments with careful software engineering to make those kinds of calculations more than just theoretically possible," said Musaelian.
"Allegro is widely applicable and is already being rapidly adopted, both in academia and industry, with applications in organic electronics, catalysts, polymers, metal alloys, coatings, batteries. We are very excited about many possibilities and technological impact of these models in the near future," said Kozinsky.
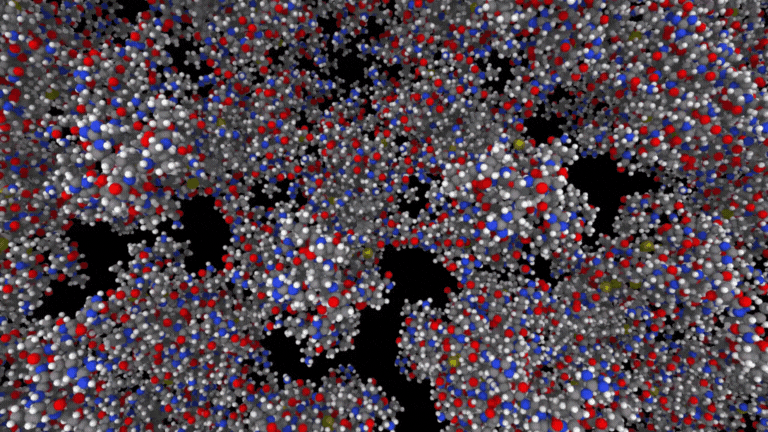
Authorship, funding, disclosures
This work was supported by the Department of Energy and the National Science Foundation through the Harvard University Materials Research Science and Engineering Center (DMR-2011754), the Office of Naval Research, and Bosch Research. Computational resources were provided by the National Energy Research Scientific Computing Center (NERSC).